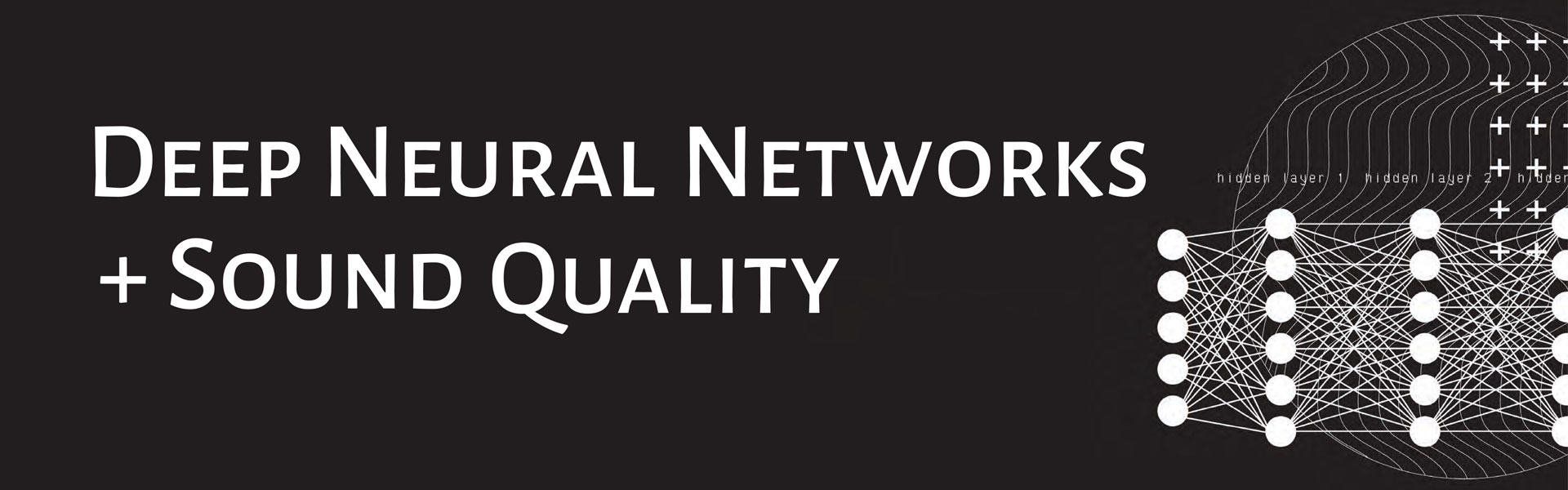
Deep Neural Networks + Sound Quality
Douglas L. Beck Au.D. and Virginia Ramachandran Au.D., Ph.D.
Introduction
Simply amplifying sound is insufficient to address speech-in-noise and other common listening and communication difficulties experienced by people with hearing loss and supra-threshold listening disorders. Specifically, to maximally understand Speech in Noise (SIN) requires an excellent signal-to-noise ratio (SNR). Despite significant advances in digital hearing aid technology and improved patient outcomes, hearing aid signal processing strategies are still constrained by physical size and power requirements (Picou, 2020).
Challenges inherent in the development of hearing aid signal processing strategies include a thorough and detailed understanding of how the brain uses audition and how the brain creates meaning from sound. Decades of hearing science research has identified auditory cues that could be used to facilitate auditory comprehension. However, what an individual brain actually does to comprehend sound and the exact meaning derived by a specific brain is not completely understood. Each human brain is unique. Although generalities can be defined and stated, the ability to understand speech in noise is a very complex process which includes hearing, hearing loss, physiology, anatomy, chemistry, knowledge, psychology, auditory processing, language and more.
By processing the auditory signal through the world’s first on-board deep neural network (DNN) of the PolarisTM platform, Oticon MoreTM uses the intricacies of the auditory signal to better process sound. Research has shown that compared to two leading premium competitor hearing aids, Oticon More has faster adaptation, more access to speech via better signal-to-noise ratio (SNR) and preferred sound quality (Man, Løve, & Garnæs, 2021; Santurette et al, 2021). Previous publications addressing Oticon More have shown an improved SNR, improved selective attention, better memory/recall, and a more representative EEG in response to the true acoustic sound scene (Santurette et al, 2020).
Deep Neural Networks
While terms such as Artificial Intelligence (AI), Machine Learning (ML), and Deep Neural Networks (DNNs) are ubiquitous, their specific meanings vary based on context, understanding, and intent. AI might be thought of as the global ability of a machine to mimic human behavior. A specific type of AI, referred to as ML, occurs when computers learn to make improved accurate predictions or decisions based on precedent.
An advanced type of ML, referred to as deep learning, occurs via DNNs. DNNs attempt to imitate how biologic brains learn. Specifically, sensory systems (vision, hearing, tactile, smell and taste) nourish the brain with vast quantities of input data. The brain detects and defines patterns among the vast data set and the brain organizes the information.
Similarly, the DNN in every Oticon More was trained on 12 million speech sound samples and real-time speech processing decisions are based on the previously achieved deep learnings. With each decision the DNN self-checks to determine the accuracy of its predication. Through “successive approximation” the output becomes increasingly accurate, all without specific instructional algorithms (Andersen et al, 2021; Bramslow & Beck, 2021). DNNs are the engine for sophisticated functions such as virtual assistants, large area facial recognition, self-driving cars, dynamic weather prediction systems, and the speech processing system in Oticon More.
Signal Processing
When DNNs are used in auditory signal processing we achieve improved listener outcomes (Andersen et al, 2021). For Oticon More, the on-board DNN, coupled with Oticon’s BrainHearingTM approach, have resulted in several measurable improvements relative to other premium hearing aid technologies (Man, Løve, & Garnæs, 2021; Santurette et al, 2021).
In a series of recent studies, the output of Oticon More devices was recorded for the left and right ears of a head and torso simulator (HATS) seated in the middle of an array of speakers. Depending on the specific study, either real-world recorded sound scenes or noise stimuli were represented via the speaker array, with target speech coming from different locations. The output of the hearing aids was used to examine the impact of the signal processing on a variety of metrics including speed of adaptation, access to speech, and sound quality.
The speed of adaptation impacts the ability of a hearing aid to act upon a signal so it can be adjusted to meet the needs of the user in a timely manner. The decision-making strategies used by a hearing aid impacts the speed with which it can identify and adjust to the environment, which impacts the SNR (and other factors) experienced by the user. Oticon More adapts to a changing sound scene 2-3 times faster than premium competitor hearing aids (Santurette et al, 2021).
Increased access to speech sounds is a requirement for improved speech understanding in complex acoustic situations. The output SNR of the studied hearing aids was calculated for target speech from the front (-15˚ azimuth) and from the side (-60˚ azimuth) in a background of noise. Under these circumstances, Oticon More was shown to have a similar SNR benefit as beamforming for signals coming from the front, while simultaneously, Oticon More demonstrated a better SNR than the competitors for speech coming from the side (Figure 1). In addition to providing more access to sound, Oticon More demonstrated overall improved SNR outcomes (Santurette et al, 2021).
Sound Quality
Sound quality is a key issue when selecting hearing aids yet is notoriously difficult to quantify. The characterization of sound quality pivots on internal abilities and factors within the observer and external factors of the perceived physical sound including softness, loudness, brightness, clarity, fullness, nearness, spaciousness, naturalness, richness/fidelity, loud-sound comfort, own voice, hearing thresholds, auditory neural synchrony, middle ear status, listening ability and much more. Thus, measurement of sound quality depends upon using carefully chosen and clearly identified measurement strategies.
The sound quality of Oticon More was evaluated against two premium competitor devices using a previously validated assessment technique, the Multi Stimulus Test with Hidden Reference and Anchor (MUSHRA), adapted for listeners with hearing loss (Beck, Tryanski & Man, 2021). Twenty-two participants with various degrees of hearing loss evaluated the sound quality of Oticon More in a blinded preference test. Stimuli consisted of a variety of sound types including café, speech in noise, and conversation with a face mask. Audio clips were recorded on the HATS after being processed by the different manufacturer hearing aids. Listeners heard the sound samples under earphones and rated the sound quality of each stimulus. When averaged across condition, Oticon More was rated highest by nearly 8 out of 10 listeners (Man, Løve, & Garnæs, 2021).
Summary
Oticon continues to build on our BrainHearing philosophy with Oticon More and its onboard DNN. In this report, the processing speed of adaptation, increased access to speech sounds and overall sound quality have been compared to two leading competitors. Oticon More demonstrated improved patient outcomes in these same measures. ■
References
- Andersen, A.H., Santurette, S., Pedersen, M.S., Alickovic, E., Fiedler, L., Jensen, J., & Behrens, T. (2021). Creating Clarity in Noisy Environments by Using Deep Learning in Hearing Aids. Seminars in Hearing, 42, 260-281.
- Beck, DL., Tryanski, D. & Man, BKL. (2021): Sound Quality and Hearing Aids. Hearing Review. August.
- Picou, E.M. (2020). MarkeTrak 10 (MT10) Survey Results Demonstrate High Satisfaction with and Benefits from Hearing Aids. Seminars in Hearing, 41(1), 21-36.
- Man B.K.L, Løve S., Garnæs M.F. (2021) Oticon More competitive benchmark. Part 2 – Clinical evidence [White paper]. Oticon
- Santurette, S., & Behrens, T. (2020). The audiology of Oticon More™ [White paper]. Oticon.
- Santurette, S., Ng, E. H. N., Juul Jensen, J., & Man K. L., B. (2020). Oticon More clinical evidence [White paper]. Oticon.
- Santurette, S., Xia, L., Ermert, C. A., & Man K. L., B. (2021). Oticon More competitive benchmark. Part 1 – Technical evidence [White paper]. Oticon.
Douglas L. Beck Au.D. earned his master's degree at the University of Buffalo (1984) and his doctorate from the University of Florida (2000). His professional career began in Los Angeles at the House Ear Institute in cochlear implant research and intraoperative cranial nerve monitoring. By 1988, he was Director of Audiology at Saint Louis University. Eight years later he co-founded an audiology and hearing aid dispensing practice in St Louis. In 1999, he became Editor-In-Chief of AudiologyOnline.com, SpeechPathology.com and HealthyHearing.com. Dr. Beck joined Oticon in 2005 as Director of Professional Relations. From 2008 through 2015, Beck was Web Content Editor for the American Academy of Audiology (AAA). In 2016 he became an adjunct Professor of Communication Disorders and Sciences at State University of New York at Buffalo (SUNYAB). In 2016, he was appointed Senior Editor for Clinical Research at the Hearing Review. In 2019, he was promoted to Vice President of Academic Sciences at Oticon Inc.
Virginia Ramachandran, Au.D., Ph.D. is Head of Audiology at Oticon, Inc. She also serves as an adjunct instructor at Wayne State University and Western Michigan University where she teaches courses in amplification. Dr. Ramachandran began her career in the field of social work.